Scraping Airlines Bots: Insights Obtained Studying Honeypot Data
Abstract
Keywords
Citation Metrics
Full Text:
PDF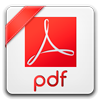
References
Amin Azad, B., Starov, O., Laperdrix, P., & Nikiforakis, N. (2020). Web runner 2049: Evaluating third-party anti-bot services. In the 17th conference on detection of intrusions and malware & vulnerability assessment (DIMVA 2020). Lisboa, Portugal. https://doi.org/10.1007/978-3-030-52683-2_7
The Bait and Switch Honeypot. (n.d.) VIEW ITEM. (Accessed: 2020-06-19)
Catakoglu, O., Balduzzi, M., & Balzarotti, D. (2016). Automatic extraction of indicators of compromise for web applications. In Proceedings of the 25th international conference on world wide web (pp. 333–343). https://doi.org/10.1145/2872427.2883056
Cheswick, B. (1992). An evening with berferd in which a cracker is lured, endured, and studied. In Proc. Winter USENIX Conference (pp. 20–24). San Francisco, CA, USA.
Chiapponi, E., Catakoglu, O., Thonnard, O., & Dacier, M. (2020). HoPLA: a Honeypot Platform to Lure Attackers. In Computer & Electronics Security Applications Rendez-vous, Deceptive security Conference (C&ESAR 2020), part of European Cyber Week. Rennes, France.
Chiapponi, E., Dacier, M., Todisco, M., Catakoglu, O., & Thonnard, O. (2020). Botnet sizes: when maths meet myths. In 1st International Workshop on Cyber Forensics and Threat Investigations Challenges in Emerging Infrastructures(CFTIC), held in conjunction with the 18th International Conference on Service-Oriented Computing (ICSOC 2020). Dubai, UAE.
Cohen, F. (2006). The use of deception techniques: Honeypots and decoys. Handbook of Information Security, 3(1), 646–655.
Comprehensive IP address data, IP geolocation API and database - IPinfo.io. (n.d.) VIEW ITEM. (Accessed: 2020-11-19)
Cosby, Donald J. (2003). 67th district court, tarrant county, texas. cause no. 067-194022-02: American airlines, inc. vs. farechase, inc VIEW ITEM. (Accessed: 2021-04-27)
Delong, M., Filiol, E., & David, B. (2019). Investigation and surveillance on the darknet: An architecture to reconcile legal aspects with technology. In ECCWS 2019 18th European conference on cyber warfare and security (p. 151).
Dietrich, S., Long, N., & Dittrich, D. (2000). Analyzing distributed denial of service tools: The shaft case. In Proceedings of the 14th Usenix conference on system administration (pp. 329–339). New Orleans, Louisiana, USA.
Djanali, S., Arunanto, F., Pratomo, B. A., Baihaqi, A., Studiawan, H., & Shiddiqi, A. M. (2014). Aggressive web application honeypot for exposing attacker’s identity. In 2014 the 1st international conference on information technology, computer, and electrical engineering (pp. 212–216). https://doi.org/10.1109/ICITACEE.2014.7065744
Endicott-Popovsky, B., Narvaez, J., Seifert, C., Frincke, D. A., O’Neil, L. R., & Aval, C. (2009). Use of deception to improve client honeypot detection of drive-by-download attacks. In International conference on foundations of augmented cognition (pp. 138–147). https://doi.org/10.1007/978-3-642-02812-0_17
Fraud prevention | detect fraud | fraud protection | prevent fraud with IPQS. (n.d.) VIEW ITEM. (Accessed: 2020-11-19)
Garg, N., & Grosu, D. (2007). Deception in honeynets: A game-theoretic analysis. In 2007 IEEE SMC information assurance and security workshop (pp. 107–113). https://doi.org/10.1109/IAW.2007.381921
Haque, A., & Singh, S. (2015). Anti-scraping application development. In 2015 international conference on advances in computing, communications and informatics (ICACCI) (p. 869-874). Kochi, India. https://doi.org/10.1109/ICACCI.2015.7275720
Higher Regional Court of Hamburg. (2009). Ryanair vs Vtours. (decision dated 28 Mai 2009, file no 3 U 191/08, ECLI:DE:OLGHH:2009:0528.3U191.08.0A)
Imperva. (2019). How bots affect airlines (Tech. Rep.). Author. Retrieved from VIEW ITEM. (Accessed: 2021-02-21)
Imperva. (2020). Imperva bad bot report (Tech. Rep.). Author. Retrieved from VIEW ITEM. (Accessed: 2021-02-21)
IpInfo.io. (August 2020). Personal communication.
Jung, J., & Sit, E. (2004). An empirical study of spam traffic and the use of DNS blacklists. In Proceedings of the 4th ACM SIGCOMM conference on internet measurement (p. 370–375). Taormina, Sicily, Italy: Association for Computing Machinery. https://doi.org/10.1145/1028788.1028838
Labrea: ”sticky” honeypot and ids. (n.d.) VIEW ITEM. (Accessed: 2021-02-21)
Laperdrix, P., Bielova, N., Baudry, B., & Avoine, G. (2020, April). Browser fingerprinting: A survey. ACM Trans. Web, 14(2). https://doi.org/10.1145/3386040
Leita, C., & Dacier, M. (2008). Sgnet: a worldwide deployable framework to support the analysis of malware threat models. In 2008 seventh European dependable computing conference (pp. 99–109). https://doi.org/10.1109/EDCC-7.2008.15
Levina, E., & Bickel, P. (2001). The earth mover’s distance is the mallows distance: Some insights from statistics. In Proceedings eighth IEEE international conference on computer vision. iccv 2001 (Vol. 2, pp. 251–256). Vancouver, Canada. https://doi.org/10.1109/ICCV.2001.937632
Li, X., Azad, B. A., Rahmati, A., & Nikiforakis, N. (2021). Good bot, bad bot: Characterizing automated browsing activity. In 2021 IEEE symposium on security and privacy (sp) (p. 17).
McKenna, S. (2016). Detection and classification of web robots with honeypots (Unpublished master’s thesis). Naval Postgraduate School, Monterey, California, USA.
Mi, X., Feng, X., Liao, X., Liu, B., Wang, X., Qian, F., . . . Liu, Y. (2019). Resident Evil: Understanding residential IP proxy as a dark service. In 2019 IEEE symposium on security and privacy (SP) (pp. 1185–1201). San Francisco, CA, USA. https://doi.org/10.1109/SP.2019.00011
Ni, D. (2019). Top 10 residential, backconnect & rotating proxies for web scraping VIEWITEM. (Accessed: 2020-08-20)
Nicomette, V., Kaaniche, M., Alata, E., & Herrb, M. (2011). Set-up and deployment of a high-interaction honeypot: experiment and lessons learned. In (Vol. 7, pp. 143–157), Springer. https://doi.org/10.1007/s11416-010-0144-2
Nunes, S., & Correia, M. (2010). Web application risk awareness with high interaction honeypots. In Actas do inforum simposio de informatica (September 2010).
Pouget, F., & Dacier, M. (2004). Honeypot-based forensics. In Auscert Asia pacific information technology security conference.
Pouget, F., Dacier, M., & Debar, H. (2003). White paper: honeypot, honeynet, honeytoken: terminological issues (Tech. Rep.Nos. EURECOM+1275), Eurecom. Retrieved from VIEW ITEM
Regional Court of Hamburg. (2008). Ryanair vs Vtours. (decision dated 28 August 2008, file no 315 O 326/08, ECLI:DE:LGHH:2008:0828.315O326.08.0A)
Samarasinghe, N., & Mannan, M. (2019a). Another look at TLS ecosystems in networked devices vs. web servers. Computers & Security, 80, 1 – 13. https://doi.org/10.1016/j.cose.2018.09.001
Samarasinghe, N., & Mannan, M. (2019b, 07). Towards a global perspective on web tracking. Computers & Security, 87, 101569. https://doi.org/10.1016/j.cose.2019.101569
Scipy.optimize Curve fit function. (n.d.) VIEW ITEM. (Accessed: 2020-07-21)
Stigler, S. M. (1989). Francis galton’s account of the invention of correlation. Statistical Science, 4(2), 73–79. https://doi.org/10.1214/ss/1177012580
Suzuki, K., Tonien, D., Kurosawa, K., & Toyota, K. (2006). Birthday paradox for multi-collisions. In M. S. Rhee & B. Lee (Eds.), Information security and cryptology – ICISC 2006 (pp. 29–40). Berlin, Heidelberg: Springer Berlin Heidelberg. https://doi.org/10.1007/11927587_5
Takemori, K., Rikitake, K., Miyake, Y., & Nakao, K. (2003). Intrusion trap system: an efficient platform for gathering intrusion-related information. In 10th international conference on telecommunications, 2003. ICT 2003. (Vol. 1, pp. 614–619 vol.1). https://doi.org/10.1109/ICTEL.2003.1191480
Thonnard, O., & Dacier, M. (2008). Actionable knowledge discovery for threats intelligence support using a multi-dimensional data mining methodology. In 2008 ieee international conference on data mining workshops (pp. 154–163). https://doi.org/10.1109/ICDMW.2008.78
Thonnard, O., Mees, W., & Dacier, M. (2009). Addressing the attack attribution problem using knowledge discovery and multi-criteria fuzzy decision-making. In Proceedings of the ACM SIGKDD workshop on cybersecurity and intelligence informatics (pp. 11–21). https://doi.org/10.1145/1599272.1599277
Tor project. (n.d.) VIEW ITEM. (Accessed: 2020-08-14)
Tzu, S. (1971). The art of war (Vol. 361). Oxford University Press, USA.
Vastel, A., Rudametkin, W., Rouvoy, R., & Blanc, X. (2020, February). FP-Crawlers: Studying the Resilience of Browser Fingerprinting to Block Crawlers. In O. Starov, A. Kapravelos, & N. Nikiforakis (Eds.), MADWeb’20 - NDSS Workshop on Measurements, Attacks, and Defenses for the Web. San Diego, United States. https://doi.org/10.14722/madweb.2020.23010
Venema, W. Z. (1992). TCP wrapper: Network monitoring, access control, and booby traps. In Usenix summer.
Von Ahn, L., Blum, M., Hopper, N. J., & Langford, J. (2003). CAPTCHA: Using hard AI problems for security. In E. Biham (Ed.), Advances in Cryptology — EUROCRYPT 2003 (pp. 294–311). Berlin, Heidelberg: Springer Berlin Heidelberg. https://doi.org/10.1007/3-540-39200-9_18